Introduction
The Human Development Index (HDI) is a statistic composite index of life expectancy, education (Literacy Rate, Gross Enrollment Ratio at different levels and Net Attendance Ratio), and per capita income indicators, which are used to rank countries into four tiers of human development. It was developed by Pakistani economist Mahbub ul Haq and has been used since 1993 by the United Nations Development Programme.
HDI aims to convey the social and economic development levels of countries beyond just economic metrics. It is used to distinguish whether a country is developed, developing or underdeveloped. HDI is also used to measure impacts of economic policies on quality of life.
However, calculating HDI can be an expensive and time consuming process, especially for developing countries with limited resources. This article examines cost effective methods and data sources that can be leveraged to calculate HDI in a budget friendly manner without compromising accuracy.
Cost Effective Data Collection Methods
Collecting quality data on life expectancy, education levels, and income can be challenging for resource strapped countries. Some cost effective data collection methods are:
Life Expectancy
- Utilize Existing Sources: Leverage life expectancy data from WHO, World Bank, UNICEF, etc. These agencies already publish statistics at the country level annually.
- Sampling Surveys: Conduct sampling surveys covering select regions/demographics instead of a full census to estimate overall life expectancy.
Mean Years of Schooling
- Use Admin Data: Use existing admin data from schools and education departments on enrollment ratios and years of schooling completed.
- Household Surveys: Conduct periodic household surveys with education level questions instead of full census.
Expected Years of Schooling
- Proxy Enrollment Ratios: Use Gross Enrollment Ratios (GER) as proxy for Expected Years of Schooling, since GER data is available annually.
- Cohort Modeling: Build a cohort model to estimate expected years of schooling from periodic household surveys instead of measuring it directly each year.
GNI Per Capita
- Use World Bank Data: Leverage GDP per capita PPP data published annually by World Bank in its World Development Indicators.
Alternative Low Cost Data Sources
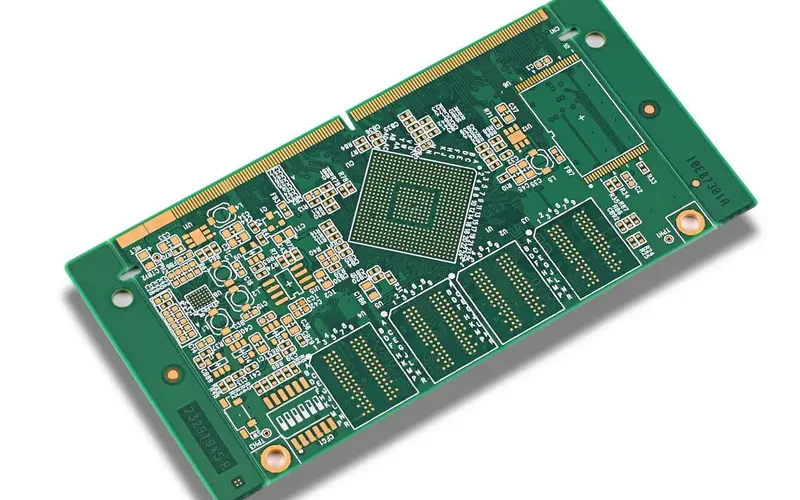
Some alternative data sources that can help estimate HDI components in a cost effective manner are:
- Mobile Phone Surveys: Collect education and income data through mobile phone surveys using random digit dialing. Cost effective way to reach a sample of population.
- Satellite Imagery: Use satellite imagery and machine learning algorithms to estimate electricity access, assets ownership, infrastructure development etc. which can proxy living standards.
- Online Activity Data: Mine data from online activites like search engines, social media, e-commerce sites etc. to gauge literacy levels, income, lifestyles etc.
- APIs and Open Data Portals: Leverage education, health, economic data available for free through government open data portals and APIs.
Optimizing Processes
Some ways to optimize HDI computation processes for efficiency are:
Automation
- Automate data collection, cleaning, analysis and report generation tasks to speed up processes and reduce human effort.
Sampling
- Use sampling techniques to gather data for only a subset instead of collecting data for the full population. Significantly reduces data collection costs.
Cloud Computing
- Leverage cloud computing platforms like AWS, GCP, Azure to run HDI computations instead of physical infrastructure. Allows scaling up and down on demand.
Open Source Software
- Deploy open source software tools for data analysis, visualization and modelling like Python, R, TensorFlow etc. to avoid licensing costs of proprietary software.
Regional Cooperation
- Partner with neighboring countries to share processes, systems and best practices to benefit from shared knowledge, resources and economies of scale.
Improving Modeling Techniques
Some ways to enhance HDI modeling techniques for better accuracy and efficiency are:
Regression Modeling
- Use multivariate regression models to predict HDI from a number of indicator variables. Allows missing variables to be estimated from correlated indicators.
Outlier Detection
- Apply outlier identification techniques to remove anomalies and smooth noisy data. Results in more robust models.
Synthetic Data Modeling
- Generate synthetic datasets to overcome limitations of small sample sizes, especially at provincial and district levels. Enables granular models.
Ensembles and Meta Learning
- Train multiple models using different samples, methodologies. Then combine them into ensemble models or use stacking/blending techniques to get more accurate predictions.
Key Takeaways
- Leverage existing data sources from international agencies instead of primary data collection
- Use sampling surveys, household surveys, school admin data instead of full census
- Deploy cloud computing, open source software and automation to optimize costs
- Cooperate with other countries to share knowledge, systems and economies of scale
- Apply advanced analytical techniques like regressions, synthetic data, ensembles/meta-learning for better insights
HDI Computation Approaches by Country Income Level
The table below summarizes the recommended approach to computing HDI in a cost effective manner basis the income level of the country:
Country Income Level | Recommended Approach |
---|---|
High Income | Use highest quality data sources, advanced modelling techniques to further enrich insights |
Upper Middle Income | Leverage wider range of indicators beyond HDI minimum, have balance of quality and cost optimization |
Lower Middle Income | Focus on finding low cost alternate data sources, limit indicators to essential HDI components |
Low Income | Prioritize automation, sampling, partnerships to minimize data costs. Accept approximations with higher error margins. |
Frequently Asked Questions
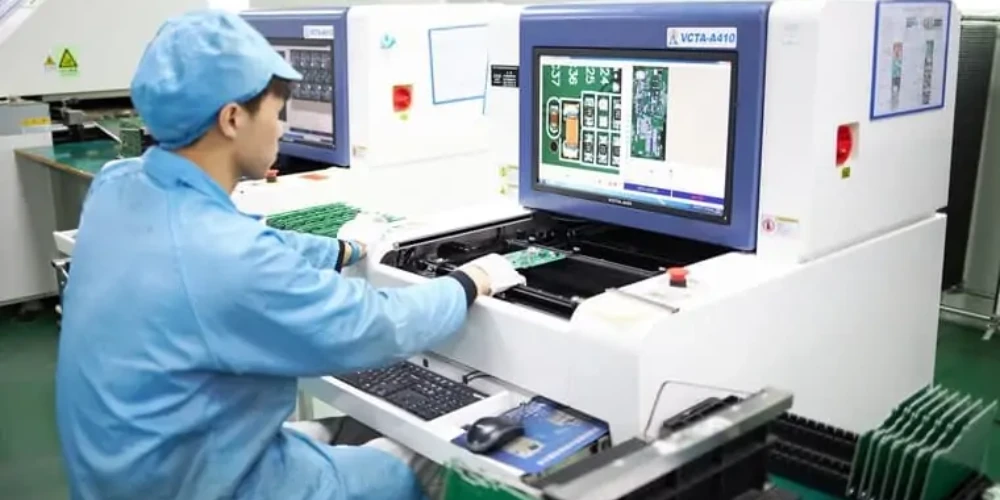
Q1. What are some limitations of using secondary data sources for HDI instead of primary data collection?
Some limitations are:
- Data may not match the definition/coverage needed
- Secondary sources may have biases, errors leading to inaccurate estimates
- Data reporting lags mean it is not fully up to date
- Granularity of data may not be available at provincial/district level
However, the cost savings often outweigh these limitations for resource constrained countries. Statistical techniques can help address biases and inaccuracies.
Q2. How can countries collaborate with each other to reduce HDI computation costs?
Countries can collaborate in the following ways:
- Share systems, applications built to automate HDI data collection and calculation
- Jointly organize household surveys across borders to gather consistent data
- Setup shared analytics infrastructure like data centres, dashboarding systems
- Exchange processes, best practices to learn from each other
- Train each other’s personnel and experts
- Create joint working groups and councils to coordinate efforts
Regional cooperation enables pooling of resources and significantly reduces costs.
Q3. Which open source tools are most useful for HDI modelling and analytics?
Some open source tools that are very useful are:
- R and Python for statistical modeling and machine learning
- TensorFlow, PyTorch, Keras for deep learning models
- Apache Spark for big data processing
- Elasticsearch for search and analytics
- Apache Airflow for workflow automation
- Grafana, Tableau, Power BI for reporting and visualizations
- Hadoop ecosystem for data storage and operations
These provide powerful capabilities at zero licensing cost.
Q4. How can we estimate education levels from sources like satellite imagery and mobile phone surveys?
Some ways are:
Satellite Imagery:
- Identify schools, educational institutes from images to estimate access
- Assess infrastructure like buildings, electricity to gauge quality
- Use night lights data to estimate literacy rates and development
Mobile Phone Surveys:
- Directly ask education level, years of schooling from respondents
- Analyze usage patterns to predict literacy levels
- Look at ownership of devices, mobile data plans as proxy for income
Advanced modeling techniques help convert these surrogate indicators into predicted education levels.
Q5. What are some best practices in sampling design and methodology to balance accuracy and cost for HDI surveys?
Some best practices are:
- Stratify sampling across geographical regions, income segments and demographics for representative coverage
- Calculate optimal sample sizes required using statistical power analysis techniques
- Leverage cluster sampling by selecting villages/towns as primary units to reduce logistics costs
- Use multi-stage sampling to limit number of primary units visited
- Leverage online and mobile phone surveys along with in-person surveys to maximize reach with minimum spend
- Periodically evaluate sampling strategy and expand/optimize design as required
Careful sampling design is key to quality results at lower survey costs.
Leave a Reply